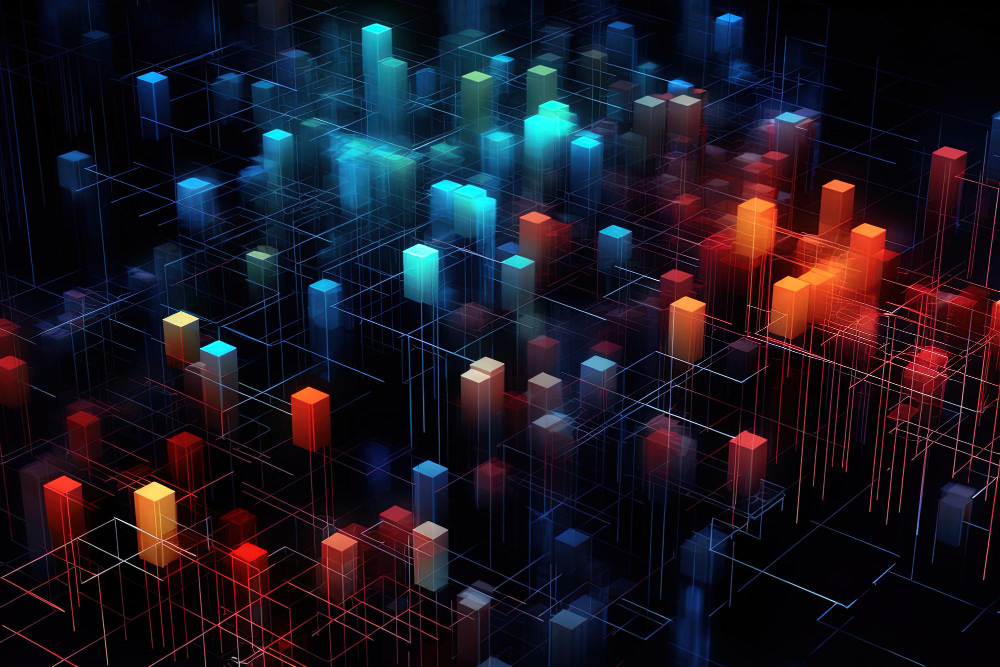
Cluster analysis may sound like a term reserved for scientists in lab coats or data analysts crunching numbers, but its applications extend far beyond the realm of academia. In fact, cluster analysis is a powerful tool used in various fields, from marketing and biology to sociology and finance. But what exactly is cluster analysis, and how does it work? Let’s embark on a journey to unravel the mysteries of cluster analysis and explore its practical applications in the real world.
An Introduction to Cluster Analysis
At its core, cluster analysis is a statistical technique used to classify objects or data points into groups, or clusters, based on their similarities or differences. The goal of cluster analysis is to identify meaningful patterns or structures within a dataset and organize it into coherent groups that share common characteristics. These clusters can then be used to gain insights into the underlying structure of the data, make predictions, or inform decision-making in various domains.
From Hierarchical to K-Means
There are several methods of cluster analysis, each with its own strengths, weaknesses, and applications. Hierarchical clustering involves organizing data points into a tree-like structure, where clusters are nested within larger clusters based on their similarity. K-means clustering, on the other hand, involves partitioning the data into a predetermined number of clusters, with each data point assigned to the cluster that minimizes the distance between them. Other methods include density-based clustering, model-based clustering, and fuzzy clustering, each offering unique approaches to clustering data.
From Market Segmentation to Genomics
Cluster analysis finds applications in a wide range of industries and fields, from market segmentation and customer profiling to genomics and bioinformatics. In marketing, cluster analysis is used to identify distinct segments of customers based on their purchasing behavior, demographics, or preferences, allowing businesses to tailor their products and marketing strategies to specific target audiences. In genomics, it is used to classify genes or proteins into functional groups based on their expression patterns or biological properties, providing valuable insights into gene function and disease mechanisms.
Navigating the Complexities
While cluster analysis can be a powerful tool for uncovering patterns and structures within data, it also comes with its challenges and considerations. One of the main challenges is determining the appropriate number of clusters, as too few clusters may oversimplify the data, while too many clusters may obscure meaningful patterns. Additionally, the choice of distance metric, clustering algorithm, and data preprocessing techniques can all influence the results of cluster analysis and require careful consideration.
Making Sense of the Clusters
Interpreting the results of cluster analysis requires a thorough understanding of the underlying data and the context in which it was collected. Researchers must carefully examine the characteristics of each cluster, assess their coherence and distinctiveness, and interpret the implications of their findings. Additionally, validating the results of cluster analysis often involves comparing them with external criteria or conducting sensitivity analyses to assess the robustness of the clustering solution.
Advancements in Cluster Analysis
As technology continues to evolve and datasets grow increasingly complex, the field of cluster analysis is also evolving to meet new challenges and opportunities. Advances in machine learning, artificial intelligence, and data mining are enabling researchers to develop more sophisticated clustering algorithms, handle larger datasets, and extract deeper insights from the data. Additionally, interdisciplinary collaborations are leading to innovative applications of cluster analysis in fields such as healthcare, finance, and environmental science.
Harnessing the Power of Cluster Analysis
In conclusion, cluster analysis is a versatile and powerful tool for organizing, analyzing, and interpreting complex datasets across a wide range of disciplines and industries. By grouping similar objects or data points into clusters, cluster analysis enables researchers and practitioners to uncover meaningful patterns, gain insights, and make informed decisions. Whether it’s identifying market segments, classifying genes, or detecting patterns in social networks, cluster analysis offers a powerful framework for understanding the structure and organization of data in the real world. So, the next time you encounter a complex dataset, consider harnessing the power of cluster analysis to unlock its hidden patterns and reveal new insights.